The digital world of machine learning and data collection is rapidly becoming a standard for many professional businesses. To uncover patterns, trends, and relationships in your data, it is necessary to learn the most efficient statistical analysis techniques. These statistical analysis methods allow companies to make data-driven decisions for their business benefit or growth.
According to Statista, the number of information and communication technology (ICT) professionals was projected to reach 55.3 million in 2020. If you’re interested in joining this field, you must know how to collect and analyze data. Statistical analysis ideas help you collect and interpret data based on various factors, ranging from descriptive analysis to cluster analysis.
Best Statistical Analysis Technique Examples
Each researcher has their preferred analysis process. Each analysis method has different qualities that allow you to make effective, advanced analyses. Below are a few of the commonly used statistical analysis techniques among research professionals.
Descriptive Statistical Analysis
More commonly known as descriptive statistics or analysis, this technique describes, shows, or constructively summarizes data points. It is the simplest statistical technique, and it uses figures and dependent variables to describe the quality of data sets. It is used for representing data but not for drawing conclusions. Tools such as tables, graphs, and charts are used to interpret data.
Associational Statistical Analysis
Associational statistical analysis is used to determine the relationship between variables. It can be used to find causation, make predictions, and can be symbolized using either positive or negative signs. This technique is very advanced. The top five tools for data analysis can help you find the linear relationships between variables.
Inferential Statistical Analysis
Inferential statistics or statistical inference is used to make inferences based on sample subjects. This allows you to make a predictive analysis about a broader population by conducting statistical tests on a few of its subjects. You may have to run many statistical tests to deduce a positive correlation.
This technique is a predictive method that saves time and allows you to make predictions and draw conclusions about a larger population in a way that cannot be done with descriptive analysis. If your results don’t reflect your research goal, you may need to consider alternative hypotheses.
Unsupervised Learning
Unsupervised learning is a technique that uses machine learning or AI algorithms to find hidden patterns in the underlying structure of a dataset without the need for human intervention. The datasets are unlabelled and grouped according to similarities using this technique.
Unsupervised learning is used when the data groups or categories are unknown and help you determine the quantitative variables of your research. You can apply this technique to three main tasks, being clustering, association, and dimensionality reduction.
Factor Analysis
Factor analysis is a data reduction technique used to reduce the number of variables into a fewer number of factors. This analysis method is one of the many qualitative data analysis techniques.
Common variance is extracted from all variables and put into a common score with factor analysis. It makes it easy to understand the underlying factors and the linear relationship between your results, giving you actionable data. Factor analysis makes it easier to interpret the central tendency of your research when you’re not overwhelmed by irrelevant information.
Cluster Analysis
Cluster analysis, also known as clustering, is an analysis process that involves grouping different objects by similarity. This method makes it easiest to determine the correlation coefficients between each group instead of dealing with random samples. You will have to compare each object to determine the similarities of each before grouping them.
Discriminant Analysis
Discriminant analysis is a statistical analysis method used by researchers to categorize observations. The dependent variables are divided into categories which makes them easier to find and better to distinguish between. This statistical method can be used to categorize variables based on their values. These variables are known as categorical variables.
Linear Regression
The linear regression model is the most commonly used predictive method. This statistical method is used to predict the value of a variable based on the value of another under the assumption that they have a linear relationship. Linear models allow you to predict a dependent variable while using an independent variable.
Simple and multiple are two types of linear regression analysis. Simple linear regression includes one predictor variable and one outcome variable. Multiple linear regression includes two or more predictor variables and one outcome variable. A comparison test can be used to compare the means of groups.
How to Collect and Analyze Data
- Determine the goal of your data collection. The first and most crucial step for data collection is determining what you hope to accomplish at the end of your research. This will help you decide on the sources, topic, method, and quantity of data you will need. Your hypothesis testing process will be much easier once you’ve established your goal.
- Choose a timeframe for the data collection. Choosing a time frame for your data collection is necessary for getting insightful results. The quantity of information you need can help you decide when to start and end your data collection.
- Choose your preferred method of data collection. Your established limitations will inform your decision on the best method for collection. You will have to consider factors such as the type of information you want to gather, the sampling distribution, your independent variables, and the quantity.
- Collect your data. Once you have specified your data collection requirements, you can begin the steps to gather your data. The best course of action is to stick to your plan at all times unless you run into any unforeseen circumstances.
- Analyze and interpret your findings. The final stage after data collection is to analyze and interpret. This can be done manually or through machine learning, although you should consider using tools or statistical software to complete it as minor mistakes can alter the accurate results.
Statistical Analysis Ideas: Top 5 Tips to Master Statistical Analysis
Statistical analysis can be pretty complex and even more so if not learned right. You will find that many people abandon it or outsource it due to getting stuck. It is important to master it before using the techniques to avoid complications. You can find below some helpful tips on how to master statistical data analysis.
Understand statistics
It’s important to learn statistics and have a deep understanding as data research is used in a wide range of industries to carry out various actions. To master statistical analysis techniques, you need to understand how statistics works, their importance, and their uses in different fields.
You can learn by reading books, doing research, and attending classes. The best online college statistics courses will allow you to learn the fundamental basics to break into the tech field.
Learn the important terms
To learn and practice in any field, you must know the technical terms. These terms are used daily and are required to understand and apply statistical analysis techniques. Statistics is a broad field and there is a long list of significant terms, but resources such as the Oxford Dictionary of Statistics can help.
Ask necessary questions
In your research and educational process, it is very important to ask professionals for guidance when you come to a misunderstanding. Not only does this give you first-hand knowledge and understanding of how statistics work, but it also keeps you from learning something the wrong way. Never feel embarrassed and ask questions at each step of the process.
Get help from a professional
When you find yourself stuck or need help, approach a professional. Although it may be faster to contact anyone who seems to understand statistics, you can be easily misled. To avoid confusion and complications, meet with a professional who has been practicing for years and understands the depths of the topics. They will help you understand faster and avoid mistakes.
Practice often
Statistics can be quite complicated and easily forgotten when not practiced. Practicing often will help you remember and get familiar with the techniques. The more you practice, the more challenges you face, and the better you get. Practicing also helps to increase the level of your data’s statistical significance and accuracy of your results.
Are Statistical Analysis Techniques Worth Learning?
Yes, statistical analysis techniques are worth learning. According to the Bureau of Labor Statistics (BLS), the average annual salary of an operations research analyst is $86,200, making this a lucrative skill to have.
Statistics are used in everyday life and knowing the best statistical analysis techniques helps you make important decisions. Accurately analyzing data can be used to influence business growth.
After learning these methods, you will never again have to wonder what is data analysis and how is it used. Your newly honed skills will allow you to give meaning to meaningless data and use it to make well-informed decisions.
Statistical Analysis Techniques FAQ
The three main types of statistical analysis are descriptive statistical analysis, inferential statistical analysis, and associational statistical analysis. Other types of statistical data analysis include predictive analysis, prescriptive analysis, and cluster analysis.
Some tools for statistical data analysis are statistical package for the social sciences (SPSS), R Foundation for Statistical Computing, Microsoft Excel, Statistical Analysis Software (SAS), and Matlab (The MathWorks).
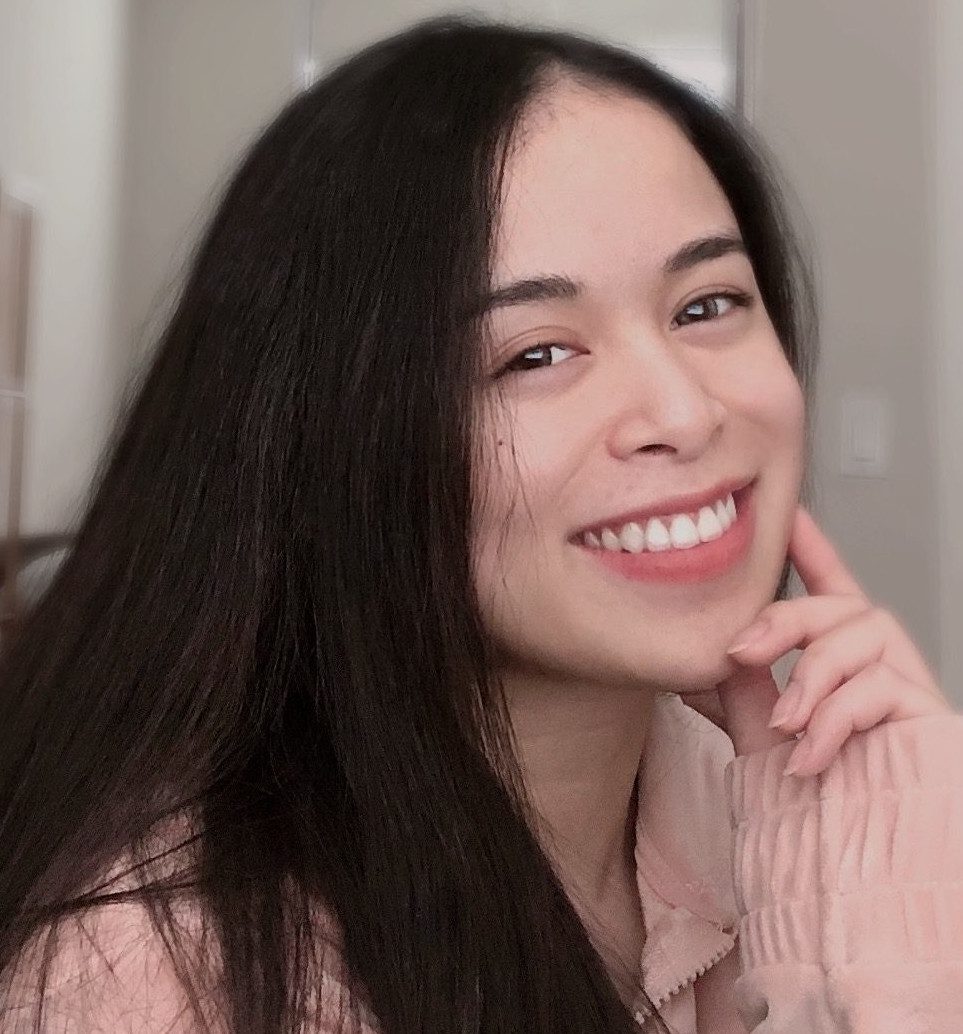
"Career Karma entered my life when I needed it most and quickly helped me match with a bootcamp. Two months after graduating, I found my dream job that aligned with my values and goals in life!"
Venus, Software Engineer at Rockbot
The five basic methods of statistical analysis are mean, standard deviation, regression, hypothesis testing, and sample size determination. You can apply different techniques to each of these methods.
The most commonly used statistical analysis measures are mean, standard deviation, F-test, t-test, analysis of variance (ANOVA), regression, and correlation. The most common doesn’t always equate to the best, so make sure to determine what measure would be the most beneficial to your data research.
About us: Career Karma is a platform designed to help job seekers find, research, and connect with job training programs to advance their careers. Learn about the CK publication.