There’s quite a lot of excitement around data science these days, with its reputation for being remunerative and future-oriented. But people often confuse it with related terms, like ‘big data’.
Both of these concepts are notoriously difficult to pin down. But we’re going to do our best to provide some clarity on the topic.
What is Data Science?
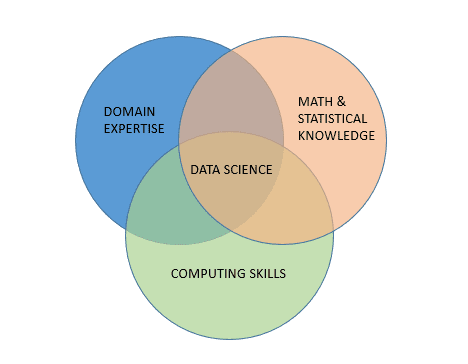
People often define data science more as the intersection of a number of other fields than as a stand-alone discipline. Most agree that it involves applying statistics and mathematics to problems in specific domains while keeping some of the insights from software engineering best practices in mind. It’s equally valid to conceptualize it as being like statistics with more coding or coding with more statistics.
Domain knowledge is extremely important, however. The kinds of data, models, techniques, and results you can expect vary widely depending on the field you’re in. You won’t be doing the same things in a startup looking to revolutionize advertising as you will be in a startup in the cryptoasset space. As a new data scientist, I spend about 101% of my waking hours learning the complicated internals of bitcoin, the blockchain, and related technologies. (If you’re wondering how I spend more than 100% of my waking hours thinking about this stuff, it’s because I also dream about it).
What Is Big Data?
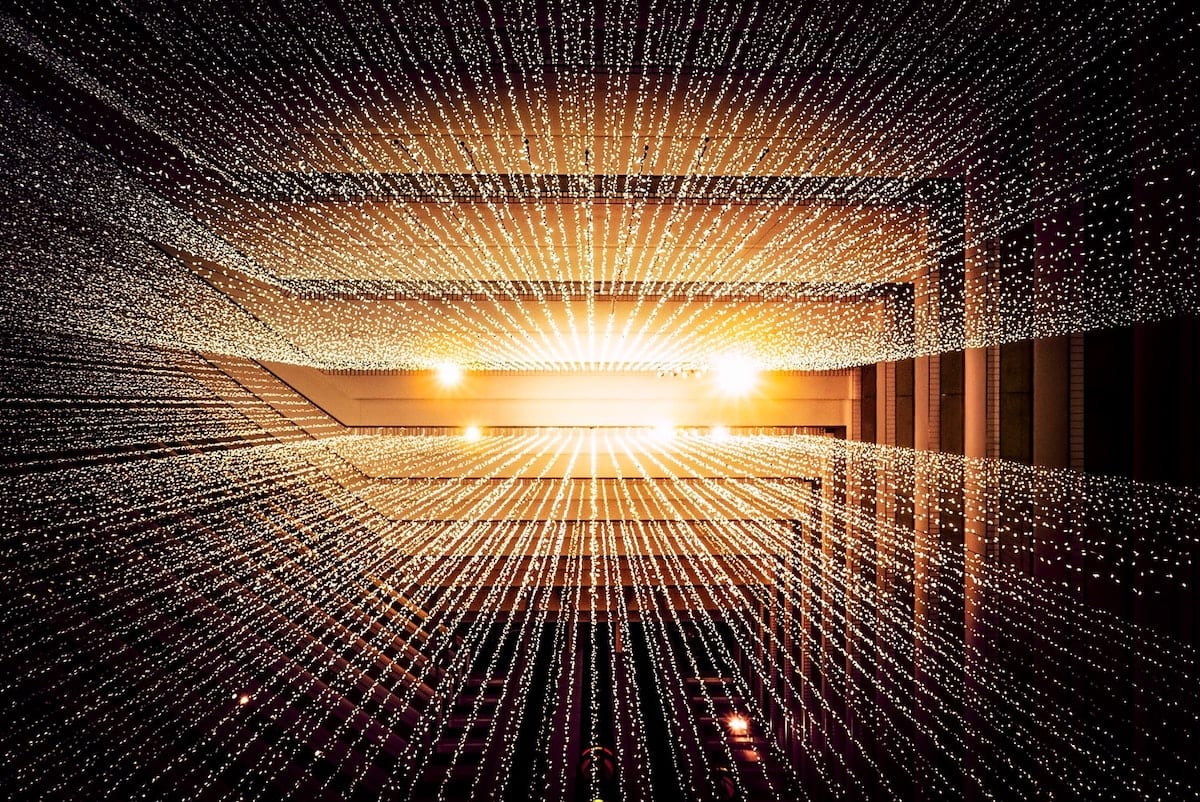
Big data is also difficult to define. At Galvanize we used the following definition: if you have more data than can fit on your local machine, you’re probably working with big data.
Another fairly common rule is that big data starts at 1 terabyte and goes up from there.
Of course, this means the definition of ‘big’ data is a moving target. If tomorrow’s desktops come with 10 terabyte hard drives, the threshold for big data will move up to that level.
But leaving aside the semantic quibbles, big data has become such an important part of the modern data science landscape that developers have come up with a whole suite of new tools specifically to deal with it, including everything from Spark to Cloud Computing. Some of my favorite Galvanize classes focused on these topics, as I think they’re going to become an ever larger portion of the data scientist’s workload.
Hopefully that clears things up a bit.
About us: Career Karma is a platform designed to help job seekers find, research, and connect with job training programs to advance their careers. Learn about the CK publication.